Threats to validity of Research Design
The books by Campbell and Stanley (1963), Cook and
Campbell (1979), and
Shadish, Cook, and Campbell, (2002) are considered seminal works in the
field of experimental design. The following write-up is based upon their books
with insertion of my examples and updated information.
Problem and Background
Experimental method and essay-writing
Campbell and Stanley point out that adherence to experimentation
dominated the field of education through the 1920s (Thorndike era) but
that this gave way to great pessimism and rejection by the late 1930s.
However, it should be noted that a departure from experimentation to
essay writing (Thorndike to Gestalt Psychology) occurred most often by
people already adept at the experimental tradition. Therefore, we must
be aware of the past so that we avoid total rejection of any method,
and instead take a serious look at the effectiveness and applicability
of current and past methods without making false assumptions.
ReplicationLack
of replicability is one of the major challenges in social science
research. After replicating one hundred psychological studies, Open
Science Collaboration (OSC) (2015) found that a large portion of the
replicated results were not as strong as the original reports in terms
of significance (p values) and magnitude (effect sizes). Specifically,
97% of the original studies reported significant results (p
< .05), but only 36% of the replicated studies yielded significant
findings. Further, the average effect size of the replicated studies
was only half of the initial studies (Mr = 0.197 vs. Mr = 0.403).
Nonetheless, the preceding problem is not surprising because usually
the initial analysis tends to overfit the model to the data. Needless
to say, a theory remains inconclusive when replicated results are
unstable and inconsistent. Multiple experimentation is more typical of
science than a one-shot
experiment! Experiments really need replication and cross-validation at
various times and conditions before the theory can be confirmed with
confidence. In the past the only option is to replicate the same
experiments over and over. Nevertheless, today the researcher is
allowed to virtually repeat the study using one single sample by
resampling. Specifically, many data mining software applications have
the features of cross-validation and bootstrap forest. In
cross-validation the data set is partitioned into many subsets and then
multiple analyses are run. In each run the model is refined by previous
"training" and thus the end result is considered a product of
replicated experiments. In a similar vein, bootstrap forest randomly
selects observations from the data and replicate the analysis many
times. The conclusion is based on the convergence of these diverse
results.
Cumulative wisdom
An interesting point made is that experiments which produce or support
opposing theories against each other probably will not have clear cut
outcomes. In fact, different researchers might observe something valid
that represents a part of the truth. Adopting experimentation in
education should not imply advocating a position incompatible with
traditional wisdom. Rather, experimentation may be seen as a process of
refining or enhancing this wisdom. Therefore, cumulative wisdom and
scientific findings need not be opposing forces.
Factors Jeopardizing Internal and External Validity
Please note that validity discussed here is in the
context of experimental design, not in the context of measurement.
- Internal validity refers specifically to whether
an
experimental treatment/condition makes a difference to the outcome or
not, and whether there is sufficient evidence to substantiate the claim.
- External validity refers to the generalizability
of the treatment/condition outcomes across various settings.
Efficacy and effectiveness
In medical studies, usually efficacy studies in
experimental settings are conducted to address the issue of internal
validity whereas effectiveness studies in naturalistic
settings (the "real" world) are employed to examine the external
validity of the claim. Usually patients in experimentation are highly
selected whereas patients in the real world are not. For example,
subjects in clinical trials usually have just the illness under study.
Patients who have multiple health conditions are excluded from the
study because those uncontrolled variables could muddle the research
results. However, in the real world it is not unusual that patients
have multiple illnesses. As a result, a drug that could work well in a
lab setting may fail in the real world. Thus, medical researchers must
take both internal validity and external validity into account while
testing the goodness of a treatment. On one hand, efficacy studies aim
to answer this question: Does the treatment work in a close
experimental environment? On the other hand, effectiveness studies
attempt to address a different issue: Does the treatment work in the
real-life situation? (Pittler & White, 1999).
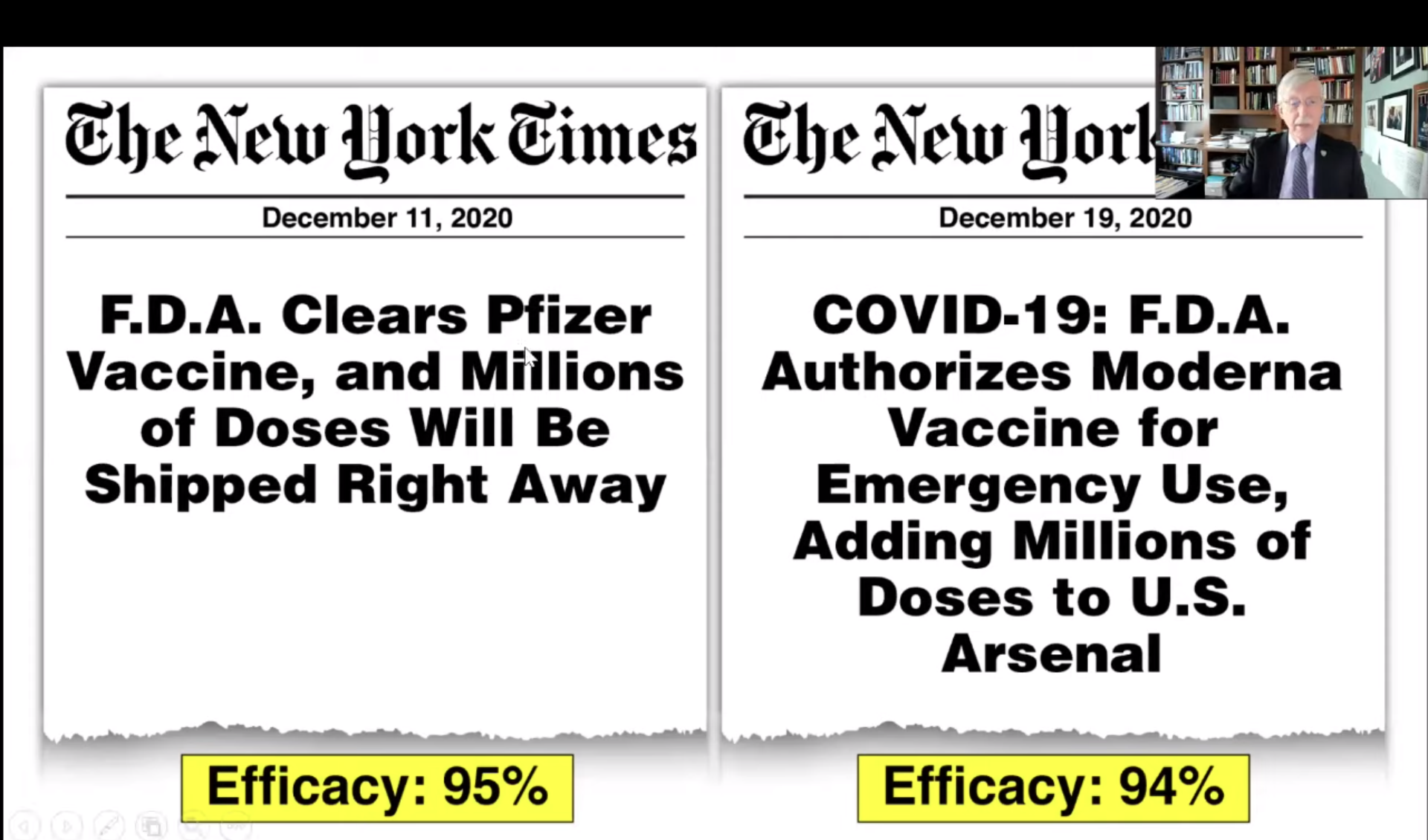
Interestingly enough, the US drug approval and monitoring processes
seem to compartmentalize efficacy and effectiveness. The US Food and
Drug administration (FDA) is responsible for approving drugs before
they are released to the market. Rigorous experiments and hard data are
required to gain the FDA's approval. But after the drugs are on the
market, it takes other agencies to monitor the effectiveness of the
drugs. Contrary to the popular belief, FDA has no authority to recall
unsafe drugs. Rather, FDA could suggest a voluntarily recall only.
Several drugs that had been approved by FDA before were re-called from
the market later (e.g. the anti-diabetic drug Avandia and pain-reliever
Vioxx). This discrepancy between the results yielded from lab tests and
the real world led to an investigation by the Institute of Medicine
(IOM). To close the gap between internal and external validity, the IOM
committee recommended that the FDA should take proactive steps to
monitor the safety of the approved drugs throughout their time on the
market (Ramsey, 2012).
Ecological validity
In recent years, the concepts of efficacy and effectiveness
is also
utilized by educational researchers (Schneider, Carnoy, Kilpatrick,
Schmidt, & Shavelson, 2007). Indeed, there is a similar concept to
"effectiveness" in educational research: ecological validity.
Educational
researchers realize that it is impossible for teachers to blocking all
interferences by closing the door. Contrary to the experimental ideal
that a good study is a "noiseless" one, a study is regarded as
ecologically valid if it captures teachers' everyday experience as they
are bombarded with numerous things (Black & Wiliam, 1998; Valli
& Buese, 2007)
Which one is more important?
Whether internal validity or external validity is more
important has been a controversial topic in the research community.
Campbell and Stanley (1963) stated that although ideally speaking a
good study should be strong in both types of validity, internal
validity is indispensable and essential while the question of external
validity is never completely answerable. External validity is concerned
with whether the same result of a given study can be observed in other
situations. Like inductive inference, this question will never be
conclusive. No matter how many new cases concur with the previous
finding, it takes just one counter-example to weaken the external
validity of the study. In other words, Campbell and Stanley's statement
implies that internal validity is more important than external
validity. Cronbach (1982) is opposed to this notion. He argued that if
a treatment is expected to be relevant to a broader context, the causal
inference must go beyond the specific conditions. If the study lacks
generalizability, then the so-called internally valid causal effect is
useless to decision makers. In a similar vein, Briggs (2008) asserted
that although statistical conclusion validity and internal validity
together affirms a causal effect, construct validity and external
validity are still necessary for generalizing a causal conclusion to
other settings.
Factors which jeopardize internal validity
- History: the specific events which occur between
the first
and second measurement. The 2008 economic recession is a good example.
Due to the budget crisis many schools cut back resources. A treatment
implemented around that period of time may be affected by a lack of
supporting infrastructure.
- Maturation:
the processes within subjects which
act
as a function of the passage of time. i.e. if the project lasts a long
period of time, most participants may improve their performance
regardless of
treatment.
- Testing: the effects of taking a test on the
outcomes of taking a second test. In other words, the pretest becomes a
form of "treatment."
- Instrumentation: the changes in the instrument,
observers, or scorers which may produce changes in outcomes.
- Statistical regression: It is also known as
regression towards the mean. This phenomenon was first discovered by
British statistician Francis Galton in the 19th century. Contrary to
popular belief, Galton found that tall parents do
not necessary have tall children.
If the parent is extremely tall, the offspring tend to closer to the
average. This pattern was re-discovered by Jewish-American psychologist
Daniel Kahneman (2011) in his study about why rebuking pilots cannot
explain flight performance. In the context of research design, the
threat of regression towards the mean is caused by the selection of
subjects on the basis of extreme scores or characteristics. If there
are forty poor students in the treatment program, it is likely that
they will show some improvement after the treatment. However, if the
students are extremely poor and thus are unresponsive to any treatment,
then it is called the floor effect.
- Selection of subjects: the biases which may result
in selection of comparison groups. Randomization (Random assignment) of
group membership is a counter-attack against this threat. However, when
the sample size is small, randomization may lead to Simpson Paradox,
which has been discussed in an earlier lesson.
- Experimental mortality: the loss of subjects. For
example, in a Web-based instruction project entitled Eruditio,
it started with 161 subjects and only 95 of them
completed the entire module. Those who stayed in the project all the
way to end may be more motivated to learn and thus achieved higher
performance. The hidden variable, intention to treat, might
skew the result.
- Selection-maturation interaction: the selection
of
comparison groups and maturation interacting which may lead to
confounding outcomes, and erroneous interpretation that the treatment
caused the effect.
- John Henry effect and Hawthorne effect:
John Henry was a worker who
outperformed a machine under an experimental setting because he was
aware that his performance was compared with that of a machine. The
Hawthorne effect is similar to John Henry effect in the sense that the
participants change their behaviors when they are aware of their role
as research subjects. Between 1924 and 32 the Hawthorne Works sponsored
a study to examine how lighting would influence productivity.
Researchers concluded that workers improved their productivity because
they were observed rather than better illumination. Hence, the
Hawthorne effect is also known as the observer effect. However, recent
research suggested that the evidence of the Hawthorne effect is scant
(Paradis & Sutlin, 2017).
Rosenthal’s
effect happens when the experimenter or the treatment-giver
unconsciously put greater expectations or extra care to the
participants. To examine how expectations could affect outcomes,
Rosenthal and Jacobson (1963) issued a Test of General Ability at an
elementary school in California. Later they randomly selected 20% of
the students and lied to the teachers that this group had unusual
potential for academic growth. Eight months later they gave a posttest
and found that those so-called gifted students outperformed other
students. One plausible explanation for this result is that those
teachers might have unnoticeably given the supposed gifted children
more personal interactions, more feedback, more approval, more positive
reinforcement.
This effect is also known as the Pygmalion Effect. Its root can be
traced back to a Cyprus mythology. Once upon time a sculptor named
Pygmalion carved a statue of a woman out of ivory. His statue was
beautiful and realistic that he wished for a bride that looked just
like his masterpiece. One day after he kissed the statue it came to
life. They fell in love and got married. This legend implies that a
great expectation can lead to a good result.
Factors which jeopardize external validity
- Reactive or interaction effect of testing: a
pretest might
increase or decrease a subject's sensitivity or responsiveness to the
experimental variable. Indeed, the effect of pretest to subsequent
tests has been empirically substantiated (Wilson & Putnam, 1982,
Lana, 1959).
- Interaction effects of selection biases and the
experimental variable
- Reactive effects of experimental arrangements: it
is difficult to generalize to non-experimental settings if the effect
was attributable to the experimental arrangement of the research.
- Multiple treatment interference: as multiple
treatments are given to the same subjects, it is difficult to control
for the effects of prior treatments.
Three Experimental Designs
To make things easier, the following will act as representations within
particular designs:
- X: Treatment
- O: Observation or measurement
- R: Random assignment
The three experimental designs discussed in this section are:
The One Shot Case Study
There is a single group and it is studied only once. A group is
introduced to a treatment or condition and then observed for changes
which are attributed to the treatment
X O
The problems with this design are:
- A total lack of manipulation. Also, the scientific
evidence is very
weak in terms of making a comparison and recording contrasts.
- There is also a tendency to have the fallacy of misplaced
precision, where the researcher engages in tedious collection of
specific detail, careful observation, testing and etc., and
misinterprets this as obtaining solid research. However, a detailed
data collection procedure should not be equated with a good design. In
the chapter on design, measurement,
and analysis, these three components are clearly distinguished from
each other.
- History, maturation, selection, mortality, and
interaction
of selection and the experimental variable are potential threats
against the internal validity of this design.
One Group Pre-Posttest Design
This is a presentation of a pretest, followed by a treatment, and then
a posttest where the difference between O1 and O2
is explained by X:
O1 X O2
However, there exists threats to the validity of the above assertion:
- History: between O1 and O2
many events may have occurred apart from X to produce the
differences in outcomes. The longer the time lapse between O1
and O2, the more likely history becomes a threat.
- Maturation: between O1 and O2
students may have grown older or internal states may have changed and
therefore the differences obtained would be attributable to these
changes as opposed to X. For example, if the US government does
nothing to the economic depression starting from 2008 and let the
crisis runs its course (this is what Mitt Romney said), ten years later
the economy may still be improved. In this case, it is problematic to
compare the economy in 2021 and that in 2011 to determine whether a
particular policy is effective; rather, the right way is to compare the
economy in 2021 with the overall (e.g. 2011 to 2021). In SPSS the
default pairwise comparison is to contrast each measure with the final
measure, but it may be misleading. In SAS the default contrast scheme
is Deviation, in which each measure is compared to the grand
mean of all measures (overall).
- Testing: the effect of giving the pretest itself
may effect the outcomes of the second test (i.e., IQ tests taken a
second time result in 3-5 point increase than those taking it the first
time). In the social sciences, it has been known that the process of
measuring may change that which is being measured: the reactive effect
occurs when the testing process itself leads to the change in behavior
rather than it being a passive record of behavior (reactivity: we want
to use non-reactive measures when possible).
- Instrumentation: examples are in threats to
validity above
- Statistical regression: or regression toward the
mean. Time-reversed control analysis and direct examination for changes
in population variability are proactive counter-measures against such
misinterpretations of the result. If the researcher selects a very
polarized sample consisting of extremely skillful and extremely poor
students, the former group might either show no improvement (ceiling
effect) or decrease their scores, and the latter might appear to show
some improvement. Needless to say, this result is midleading, and to
correct this type of misinterpretation, researchers may want to do a
time-reversed (posttest-pretest) analysis to analyze the true treatment
effects. Researchers may also exclude outliers from the analysis or to
adjust the scores by winsorizing the means (pushing the outliers
towards the center of the distribution).
- Others: History, maturation, testing,
instrumentation interaction of testing and maturation, interaction of
testing and the experimental variable and the interaction of selection
and the experimental variable are also threats to validity for this
design.
The Static Group Comparison
This is a two group design, where one group is exposed to a treatment
and the results are tested while a control group is not exposed to the
treatment and similarly tested in order to compare the effects of
treatment.
Threats to validity include:
- Selection: groups selected may actually be
disparate prior to any treatment.
- Mortality: the differences between O1
and O2
may be because of the drop-out rate of subjects from a specific
experimental group, which would cause the groups to be unequal.
- Others: Interaction of selection and maturation
and interaction of selection and the experimental variable.
Three True Experimental Designs
The next three designs discussed are the most strongly recommended
designs:
The Pretest-Posttest Control Group Design
This designs takes on this form:
This design controls for all of the seven threats to validity described
in detail so far. An explanation of how this design controls for these
threats is below.
- History: this is controlled in that the general
history events which may have contributed to the O1
and O2 effects would also produce the O3
and O4
effects. However, this is true if and only if the experiment is run in
a specific manner: the researcher may not test the treatment and
control groups at different times and in vastly different settings as
these differences may influence the results. Rather, the researcher
must test the control and experimental groups concurrently.
Intrasession history must also be taken into account. For example if
the groups are tested at the same time, then different experimenters
might be involved, and the differences between the experimenters may
contribute to the effects.
In this case, a possible counter-measure is the
randomization of
experimental conditions, such as counter-balancing in terms of
experimenter, time of day, week and etc.
- Maturation and testing: these are controlled in
the sense that they are manifested equally in both treatment and
control groups.
- Instrumentation: this is controlled where
conditions control for intrasession history, especially where the same
tests are used. However, when different raters, observers or
interviewers are involved, this becomes a potential problem. If there
are not enough raters or observers to be randomly assigned to different
experimental conditions, the raters or observers must be blind to the
purpose of the experiment.
- Regression: this is controlled by the mean
differences regardless of the extremely of scores or characteristics,
if the treatment and control groups are randomly assigned from the same
extreme pool. If this occurs, both groups will regress similarly,
regardless of treatment.
- Selection: this is controlled by randomization.
- Mortality: this was said to be controlled in this
design. However, unless the mortality rate is equal in treatment and
control groups, it is not possible to indicate with certainty that
mortality did not contribute to the experiment results. Even when even
mortality actually occurs, there remains a possibility of complex
interactions which may make the effects drop-out rates differ between
the two groups. Conditions between the two groups must remain similar:
for example, if the treatment group must attend the treatment session,
then the control group must also attend sessions where either no
treatment occurs, or a "placebo" treatment occurs. However, even in
this there remains possibilities of threats to validity. For example,
even the presence of a "placebo" may contribute to an effect similar to
the treatment, the placebo treatment must be somewhat believable and
therefore may end up having similar results!
The factors described so far affect internal validity. These factors
could produce changes, which may be interpreted as the result of the
treatment. These are called main effects, which have been
controlled in this design giving it internal validity.
However, in this design, there are threats to external
validity (also called interaction effects
because they involve the treatment and some other variable the
interaction of which cause the threat to validity). It is important to
note here that external validity or generalizability always turns out
to involve extrapolation into a realm not represented in one's sample.
In contrast, internal validity are solvable by the logic of
probability
statistics, meaning that we can control for internal validity based on
probability statistics within the experiment conducted. On the other
hand, external validity or generalizability can not logically occur
because we can't logically extrapolate to different settings. (Hume's
truism that induction or generalization is never fully justified
logically).
External threats include:
- Interaction of testing and X: because the
interaction
between taking a pretest and the treatment itself may effect the
results of the experimental group, it is desirable to use a design
which does not use a pretest.
- Interaction of selection and X: although selection
is controlled for by randomly assigning subjects into experimental and
control groups, there remains a possibility that the effects
demonstrated hold true only for that population from which the
experimental and control groups were selected. An example is a
researcher trying to select schools to observe, however has been turned
down by 9, and accepted by the 10th. The characteristics of
the 10th
school may be vastly different than the other 9, and therefore not
representative of an average school. Therefore in any report, the
researcher should describe the population studied as well as any
populations which rejected the invitation.
- Reactive arrangements: this refers to the
artificiality of the experimental setting and the subject's knowledge
that he is participating in an experiment. This situation is
unrepresentative of the school setting or any natural setting, and can
seriously impact the experiment results. To remediate this problem,
experiments should be incorporated as variants of the regular
curricula, tests should be integrated into the normal testing routine,
and treatment should be delivered by regular staff with individual
students.
Research should be conducted in schools in this manner: ideas for
research should originate with teachers or other school personnel. The
designs for this research should be worked out with someone expert at
research methodology, and the research itself carried out by those who
came up with the research idea. Results should be analyzed by the
expert, and then the final interpretation delivered by an intermediary.
Tests of significance for this design: although this design
may be
developed and conducted appropriately, statistical tests of
significance are not always used appropriately.
- Wrong statistic in common use: many use a t-test by
computing two
ts, one for the pre-post difference in the experimental group and one
for the pre-post difference of the control group. If the experimental
t-test is statistically significant as opposed to the control group,
the treatment is said to have an effect. However this does not take
into consideration how "close" the t-test may really have been. A
better procedure is to run a 2X2 ANOVA repeated measures, testing the
pre-post difference as the within-subject factor, the group
difference as the between-subject factor, and the interaction
effect of both factors.
- Use of gain scores and covariance: the most used test is
to compute pre-posttest gain scores for each group, and then to compute
a t-test between the experimental and control groups on the gain
scores. In addition, it is helpful to use randomized "blocking" or
"leveling" on pretest scores because blocking can localize the
within-subject variance, also known as the error variance. It is
important to point out that gain scores are subject to the ceiling and
floor effects. In the former the subjects start with a very high
pretest score and in the latter the subjects have very poor pretest
performance. In this case, analysis of covariance (ANCOVA) is usually
preferable to a simple gain-score comparison.
- Statistics for random assignment of intact classrooms to
treatments: when intact classrooms have been assigned at random to
treatments (as opposed to individuals being assigned to treatments),
class means are used as the basic observations, and treatment effects
are tested against variations in these means. A covariance analysis
would use pretest means as the covariate.
The Soloman Four-Group Design
The design is as:
R |
O1 |
X |
O2 |
R |
O3 |
|
O4 |
R |
|
X |
O5 |
R |
|
|
O6 |
In this research design, subjects are randomly assigned into four
different groups: experimental with both pre-posttests, experimental
with no pretest, control with pre-posttests, and control without
pretests. In this configuration, both the main effects of testing and
the interaction of testing and the treatment are controlled. As a
result, generalizability is improved and the effect of X is
replicated in four different ways.
Statistical tests for this design: a good way to test the
results is to
rule out the pretest as a "treatment" and treat the posttest scores
with a 2X2 analysis of variance design-pretested against unpretested.
Alternatively, the pretest, which is a form of pre-existing difference,
can be used as a covariate in ANCOVA.
The Posttest-Only Control Group Design
This design is as:
This design can be viewed as the last two groups in the
Solomon 4-group design. And can be seen as controlling for testing as
main effect and interaction, but unlike this design, it doesn't measure
them. But the measurement of these effects isn't necessary to the
central question of whether of not X did have an effect. This
design is appropriate for times when pretests are not acceptable.
Statistical tests for this design: the most simple form
would be the
t-test. However, covariance analysis and blocking on subject variables
(prior grades, test scores, etc.) can be used which increase the power
of the significance test similarly to what is provided by a pretest.
Discussion on causal inference and generalization
As illustrated above, Cook and Campbell devoted much efforts to
avoid/reduce the threats against internal validity (cause and effect)
and external validity (generalization). However, some widespread
concepts may also contribute other types of threats against internal
and external validity.
Some researchers downplay the importance of causal inference
and assert
the worth of understanding. This understanding includes "what," "how,"
and "why." However, is "why" considered a "cause and effect"
relationship? If a question "why X happens" is asked and the answer is
"Y happens," does it imply that "Y causes X"? If X and Y are correlated
only, it does not address the question "why." Replacing "cause and
effect" with "understanding" makes the conclusion confusing and
misdirect researchers away from the issue of "internal validity."
Some researchers apply a narrow approach to "explanation."
In this
view, an explanation is contextualized to only a particular case in a
particular time and place, and thus generalization is considered
inappropriate. In fact, an over-specific explanation might not explain
anything at all. For example, if one asks, "Why Alex Yu behaves in that
way," the answer could be "because he is Alex Yu. He is a unique human
being. He has a particular family background and a specific social
circle." These "particular" statements are always right, thereby
misguide researchers away from the issue of external validity.
Reference
- Black, P., & Wiliam, D. (1998). Assessment and
classroom learning. Assessment in Education: Principles, Policy and
Practice, 5, 7-74.
- Briggs, D. C. (2008). Comments on Slavin: Synthesizing
causal inferences. Educational Researcher, 37, 15-22.
- Campbell, D. & Stanley, J. (1963). Experimental and
quasi-experimental designs for research. Chicago, IL: Rand-McNally.
- Cook, T. D., & Campbell, D. T. (1979). Quasi-experimentation:
Design and analysis issues for field settings. Boston, MA: Houghton
Mifflin Company.
- Cronbach, L. (1982). Designing evaluations of
educational and social programs. San Francisco: Jossey-Bass.
- Kahneman, D. (2011). Thinking
fast and slow. New York, NY: Farrar, Straus, and Giroux.
- Open Science Collaboration. (2015). Estimating the reproducibility of psychological science. Science, 349(6251). doi: 10.1126/science.aac4716. Retrieved from http://science.sciencemag.org/content/349/6251/aac4716
- Paradis, E., & Sutkin, G. (2017). Beyond a good story:
from Hawthorne Effect to reactivity in health professions education
research. Medical Education, 51(1): 31-39.
- Pittler, M. H., & White, A. R. (1999). Efficacy and
effectiveness. Focus on Alternative and Complementary Therapy, 4,109–10.
Retrieved from
http://www.medicinescomplete.com/journals/fact/current/fact0403a02t01.htm
- Ramsey, L. (2012, May 2). U.S. needs to expand monitoring
after drug approval. PharmPro. Retrieved from http://www.pharmpro.com/news/2012/05/us-needs-to-expand-monitoring-after-drug-approval/
- Rosenthal, R., &. Jacobson, L. (1963). Teachers' expectancies: Determinants of pupils' IQ gains. Psychological Reports, 19, 115-118.
- Schneider, B., Carnoy, M., Kilpatrick, J. Schmidt, W. H.,
& Shavelson, R. J. (2007). Estimating causal effects using
experimental and observational designs: A think tank white paper.
Washington, D.C.: American Educational Research Association.
- Shadish, W. R., Cook, T. D., & Campbell, D. T. (2002). Experimental
and quasi-experimental designs for generalized causal inference.
Boston, MA: Houghton Mifflin.
- Valli, L., & Buese, D. (2007). The changing roles of
teachers in an era of high-stakes accountability. American
Education Research Journal, 44, 519-558.
Go up to the main menu
|
|