Treatment
effectiveness evaluation,
instrument validation
and performance appraisal
|
Introduction
Psychological and educational research often is focused on
treatment outcomes. Appropriate interpretation of treatment outcomes
is based primarily on:
- Studying the efficacy of treatment
- Establishing the validity and reliability of
instrumentation,
- Obtaining subjects who represent a normal cross-section of the
desired population to ensure accurate performance appraisal.
However, in many quasi-experimental and non-experimental settings
where experimental control is lacking, often each aspect mentioned
above is studied simultaneously. This convolutes the focus of
research as well as comprises the interpretation of results. For
example, in research on Web-based instruction, test results are often
used as an indicator of both the quality of instruction and the
knowledge level of students. In addition, pilot studies are
implemented to examine the usefulness of the treatment program and to
validate the instrument based upon the same pilot results.
To address this misapplication of methods, this article discusses
the differentiation of the objectives of treatment effectiveness
evaluation, instrument validation, and performance appraisal. In
addition, it points out the pitfalls of circular dependency, proposes
the rectification of the problems inherent in circular dependency,
and presents recommendations for the application of appropriate
research methods.
Further, a survey result was reported to substantiate these
widespread misconceptions. The data were collected via the Internet
by announcing the online survey to six different ListServ groups and
Newsgroups. The survey consists of five multiple-choice questions,
which are related to the functions of treatment effectiveness
evaluation, instrument validation, and performance appraisal (see
Appendix). Thirty-four graduate students, who had taken on average
4.9 undergraduate and graduate statistics courses, responded to the
survey. The respondents span across twenty-five institutions in six
continents/regions (North America, South America, Europe, Asia,
Africa, New Zealand), twenty-two different undergraduate majors (e.g.
chemistry, English, electrical engineering, history, psychology) and
eighteen different graduate majors (e.g. biology, communication,
computer science, education, finance, industrial engineering,
statistics). Within the United States, the respondents came from
eight different states. On the average, the participants answered 1.6
questions correctly.
Consequences of the
confusion
Researchers may be confused by the function of specific aspects in
studies of treatment outcomes, instrument reliability and validity,
as well as subjects¹ ability. Table 1 summarizes the differences
of the three functions. Confusing these differences among a study's
treatment, instruments and subjects may lead to grave
consequences.
Target
|
Type
|
Objective
|
Source of variation
|
Sample size determination
|
Treatment
|
Treatment effectiveness
evaluation
|
To evaluate the effectiveness of a treatment such as an
instructional module
|
Treatment effect and experimental errors
|
Power, beta,
effect size, alpha
|
Instrument
|
Instrument validation
|
To evaluate the reliability and validity of an instrument
such as a test or a survey
|
Instrument items
|
Stability
|
People
|
Performance/
Attitude appraisal
|
To evaluate the performance or/and the attitude of
respondents
|
Subjects' ability
|
None
|
Test-retest reliability and pre-post
difference
As an example, a common occurrence in the field follows: A
researcher administers an instrument to a group of subjects as a
pretest- treatment - posttest. The correlation between the two
measurements is then examined in an attempt to estimate the
test-retest reliability instead of treatment effectiveness. Indeed, a
dependent t-test should be performed to compute the pre-post
difference, because the source of variation is due to factors other
than the instrument. However, in instrument validation, the source of
variation is the instrument itself (e.g. poorly written items) while
in studying treatment effectiveness, the source of variation is the
treatment effect and the experimental errors. The consequence here is
manifested in the interpretation of the results from such a study:
that the instrument is either valid or invalid, and the treatment
effect either supports or does not support the hypothesis. No matter
the interpretation, the method used is inappropriate, and therefore
any discussion would be invalid.
The survey results indicate that this confusion exists though it
may not be widespread. Question 1 was used to test the subjects¹
ability to distinguish test-retest reliability from pre-post
differences. Thirty-two percent of the respondents failed to
differentiate the two concepts.
Sample size determination
Further confusion of studying treatment effectiveness and
instrument validation is manifested in sample size requirement. In
our teaching, consulting, and research experience, many students and
researchers, after calculating a desirable sample size using power
analysis, use the suggested sample size or less to run pilot studies
for instrument validation. Apparent in this act is the failure to
distinguish the sample size requirement in studying treatment
effectiveness from that in instrument validation. For example, power
is the probability of correctly rejecting the null hypothesis. Thus,
power analysis is inherent in studying treatment effectiveness where
dependent and independent variables are present. Moreover, hypothesis
testing alone does not reveal how likely the result can be replicated
in other studies (Thompson, 1996). In other words, stability across
samples, which is a focus of instrument validation, is not an issue
in sample size determination for studying treatment
effectiveness.
In contrast, instrument validation requires no distinction between
dependent and independent variables, and there is no null hypothesis
to be rejected. The sample size criterion for instrument validation
is stability rather than power. To be specific, the larger the sample
size, the more likely that the instrument can be applied to different
samples. To achieve this stability, a very large sample size is
necessary. For example, in factor analysis, which is a procedure of
loading items into latent constructs, 300 subjects are considered a
small sample (Wolins, 1995). It is not unusual for a test developer
to use as many as 5000 subjects for instrument validation (Potter,
1999). But a treatment effectiveness study with 300-5000 subjects may
be considered being overpowered in power analysis. It is apparent,
then, that using the results of a single power analysis to determine
sample size to study treatment efficacy and instrument validation
becomes problematic, and may lead to erroneous, yet over-confident
conclusions.
Question 2 and 3 were used to test the subjects¹ knowledge on
sample size determination. In Question 2, Seventy-six percent of
respondents were confused by the criteria of determining sample size
for treatment effectiveness study and instrument validation.
Eight-two percent of responses to question 3 were incorrect. It is
evident that the majority did not understand the purpose of power
analysis.
Performance/attitude appraisal is less problematic. In appraisals,
the conclusion is made to individuals. Even if there is only one
subject, the judgment is still valid to that particular person, and
therefore sample size determination is not needed.
Circular dependency
Last but not least, this confusion may lead to the logical fallacy
of circular dependency. There are two aspects of circular dependency
in classical test theory. The first is the circular dependency
between the theoretical constructs and the data; the second is the
circular dependency between the instrument and the subjects.
First, when Cronbach and Meehl (1955) proposed the concept of
construct validity, they maintained that hypothetical constructs
drive the nature of data collection. For instance, when we have a
theory about "social presence on the Internet," the questions and the
possible choices for answers in the instrumentation will be framed
within the theoretical model. In turn, the data resulting from the
administration of the instrument are then used to revise the theory
itself. Further, in studying treatment effectiveness, the treatment
is developed based on the theory. In turn, the data are used to
confirm or disconfirm the treatment effectiveness, and eventually,
the theory behind the treatment. This circular dependency may be
viewed as a positive feedback loop if the theory and the treatment
are continuously refined by appropriately analyzing data. However,
what if the research starts with terribly incorrect theoretical
constructs? In this case, the data may give the right answer to the
wrong question.
Embedded within the first circular dependency is as second: Fan
(1998) pointed out that in classical test theory, the quality of an
instrument is evaluated based upon the tester responses. At the same
time, the statistics of examinees are dependent on the quality of the
test items. In other words, in studying instrument validity and
reliability, test responses used to validate an instrument are also
dependent upon a certain degree of the appropriate construction of
that instrument. But what if the initial instrument is terribly
constructed, which may be resulted from a terribly misconceived
construct? The two types of circular dependency are illustrated in
Figure 1:
Figure 1. Two types of circular dependency
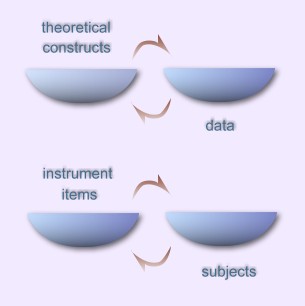
These two types of circular dependency are amplified when the
three types of evaluation are not clearly delineated and are
mistakenly run in parallel. The relationship between the subjects and
the instrument, as well as that between the instrument and the
treatment can be circular: The instrument is validated by the user
input, treatment efficacy determined by the instrument results, and
the final results used to determine the quality of students. By the
same token, the usefulness of the treatment is judged by the
learners' test performance, and later students' abilities are
measured by how much they have learned from the treatment, as
indicated by the test scores. To rectify this situation, our proposed
guideline is simple: Researchers must know two before knowing the
third.
Rectification of the inherent
problems in circular dependency
Because of the inter-dependency among the treatment, the
instrument, and the subjects, certain assumptions must be made or
certain knowledge must be obtained in order to justify an assertion
to either one of them:
- Given that the treatment is effective and the instrument is
valid, the evaluator is able to make a judgment about students'
knowledge
- Given that the treatment is effective and the subjects have
the requisite characteristics, the evaluator can find out whether
the instrument is valid or not.
- Given that the learners are ready to learn and the instrument
is valid, the evaluator can determine whether the treatment is
effective or not.
In other words, one must know or assume the quality of at least
two elements in order to unveil the third unknown. Therefore,
problems arise when there are two or more unknown elements. When all
of them are unknown, no conclusion can be made to the treatment, the
instrument, or the subject. In the survey, question 5 addresses this
problem. Only nine percent of respondents gave the correct
answer.
Many researchers commit a common logical fallacy by asserting that
"If the treatment works, the test scores are high. Thus, if the test
scores are high, then the treatment works." The notation of this
logical fallacy is: "If P then Q, therefore if Q then P." The first
statement (If P then Q), which is called "Modus ponens" (Hurley,
1988), is a valid inference. But the second one (if Q then P) is
considered the fallacy of affirming the consequence (Kelley, 1998).
Put it in a concrete example: The statements that "if it rains, the
ground is wet. If the ground is wet, it rains" are considered
invalid. To infer from Q to P (a wet ground to raining), one must
rule out other plausible rival explanations such as the city
custodians are cleaning the street, an underground water pipe
explodes, and so on. In other words, the fallacy of affirming the
consequence occurs when "ruling out" procedures are absent. By the
same token, in research it is dangerous to infer from the result (Q)
such as test scores to the cause (P) without ruling out competing
explanations. In the survey, question 4 tests the subjects¹
knowledge of logical fallacy. Fifty-six percent of participants
failed to give the right answer.
The following scenarios demonstrate how competing explanations
create uncertainties.
Scenario 1
If the evaluator knows nothing or very little about the treatment
and the instrument, but he is positive that the subjects are students
who are ready to learn the content, what could be inferred from a low
mean score? Figure 2 pictorially illustrates this scenario:
Figure 2. The treatment and instrument qualities are
unknown.
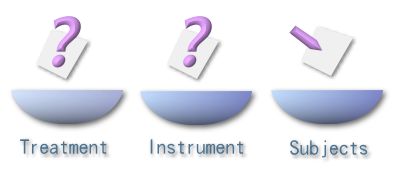
In this scenario, there are three possible explanations: either
the treatment is very poor and thus the students failed to acquire
the knowledge, the test is poorly written and thus are incapable of
reflecting their knowledge, or both. Since there are several
competing explanations, any inference made from the result to the
cause would be invalid.
Bias in Test of Economic Literacy (TEL) is a good example to
elucidate the above scenario. TEL is a validated instrument and
widely used in many research studies for assessing the knowledge
level of economics. Using Differential Item Functioning (DIF),
Walstad, W. B. and Denise (1997) found that some items in TEL are
biased against female students even if the men and women who took the
exam have the same skill levels in economics. Without this knowledge
about the instrument, the gender difference may be mistakenly
attributed to the treatment rather than to the instrument. This
finding regarding TEL is built upon the fact that the skill level of
one group is comparable to that of the other. Different groups tend
to respond differently to the same item because of cognitive
abilities and other non-biased factors. Thus, understanding the
instrument and the subjects plays a crucial role in unmasking the
nature of this gender effect.
Scenario 2
If the evaluator knows nothing or very little about the treatment
and the students, but he adopts a validated instrument, what does a
low average score of the students mean? Figure 3 illustrates this
scenario:
Figure 3. The treatment and subjects' qualities are
unknown.
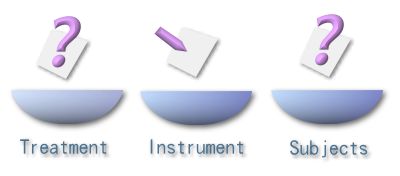
There are four possibilities: the treatment is ineffective, the
treatment is not well-implemented, the students are not ready, or
both of the above. When a treatment is tested, it is usually assumed
that the treatment is properly implmented and thus the conclusion can
be directly drawn to the quality of the treatment. Sechrest et al.
(1979) warned that the strength and integrity of the treatment may
bias the result. For example, when a new drug was introduced, the
dosage might be mild and therefore the effect was undetected. Also,
when a therapy had just been developed recently, its practitioners
might be inexperienced as opposed to the practitioners of the rival
treatment. Therefore, Sechrest et al asserted that conclusions about
whether a treatment is effective cannot be reached without the full
knowledge of how strong and well-implemented the treatment was.
The low scores could also be explained by the problem in
measurement. It is not uncommon that a low Cronbach Alpha
coefficient, which is an indicator of internal consistency, occurs
when a nationally accepted test is administered to a local group. If
the test is too difficult to a sub-standard local group, random
guessing to difficult items would lead to a low Cronbach Alpha
coefficient.
Scenario 3
If the researcher has no knowledge about the learners, nor the
test reliability or validity, but he employs a well-developed
technology-based educational product, how could he interpret a low
average test score? Figure 4 illustrates this scenario.
Figure 4. The instrument and subjects' qualities are
unknown.
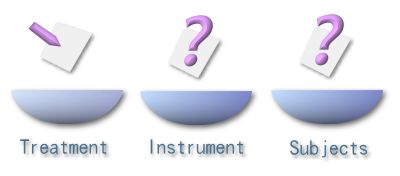
In this scenario, the outcome might be caused by the problems of
the students (the lack of basic skills or the fear of using
technology), the problems of the instrument (low reliability or
insufficient validity), or both of them. If one uses a well-developed
treatment and a validated instrument, there will be less
uncertainties in the evaluation. In this case, a quantitative study
could be applied immediately. However, educational researchers
usually develop a new treatment and a customized instrument. Also, it
is not unusual that a self-made test is used to evaluate both the
treatment and the students concurrently. Inevitably, this approach
risks circular dependency or false assumptions. Conclusions such as
"the treatment works because the participants have performance gain.
The participants improved their skill because of the treatment"
suffer serious logical fallacies.
Recommendations
The following procedures are recommended for reducing the risk of
making an invalid evaluation:
Understanding subjects
Over-estimation or under-estimation of the learners' ability and
readiness may lead to a conclusion of an ineffective treatment or an
invalid instrument. In the context of psychotherapy, Dickson (1975)
asserted, "the behavior in question must be related to client
baselines, situational expectations, and peer performances. Only
after this information is gathered should intervention take place."
(p.379) This principle can be applied to educational psychology. It
is advisable for the evaluator to conduct an initial survey to the
target audience. The assessment should be qualitative in nature. The
purpose is to understand the need and readiness of the subjects. At
this point numeric data may not be helpful to gain the insight
pertaining to the subjects.During this process, the goal is not only
to find out how much the students have already known, but also how
much they do not know.
It is a common practice that a pretest is administered prior to
the treatment. However, usually the purpose of the pretest is not to
understand the subjects. Rather it is directly applied to studying
treatment effectiveness. i.e. The pretest scores is used as a
covariate or the pretest-posttest difference is computed in a
dependent t-test. To avoid threats against the validity of experiment
such as the ceiling effect and regression to the mean, high and low
pretest scores are simply thrown out. Very few researchers ask these
questions: "Why are some people under-achievers and some
over-achievers? Is it possible that those so-called 'over-achievers'
are 'normal' but all the rest are substandard students who are not
ready for the treatment?"
Instrumentation
It is a common practice that evaluators use another validated
instrument as a reference for developing the customized instrument,
and also run several pilot studies to revise the instrument. However,
the researcher should be aware that how the theoretical constructs
are formulated dictates how the instrument is written. Observing and
interviewing subjects in an unstructured fashion may help the
researcher to discover new constructs and lead the study in a new
direction. As Messick (1980, 1988) pointed out, construct validation
is a never-ending process. New findings or new crediable assumptions
may lead to a change in construct interpretation, theory, or
measurement.
Refining treatment
The developer should use another well-established treatment as a
model for developing the new treatment, and beta test the treatment
using the think-aloud protocol (Ericsson, 1993; Ericsson & Smith,
1991). The think aloud protocol, also known as the mental protocol
and the concurrent protocol, is a data collection technique in which
the subject verbally expresses whatever he is thinking when he
performs a task. This technique is particularly useful when the
treatment involves complex mental processing.
As mentioned before, a misconceived theoretical construct could
lead to a treatment addressing the wrong research question and an
instrument evaluating the misdirected target. A think aloud protocol
can help the researcher to gain insight of a complex mental process
and thereby develop appropriate constructs. For instance, in a study
regarding teaching children using object-oriented languages to
program LEGO-made robots (Instructional Support Group, 1999), the
objective of the treatment is to enhance problem solving skills. In
this context, the specific problem solving skill is defined as the
ability of modularizing a task and restructuring the relationships
among objects (components) to form a coherent and functioning model
(Kohler, 1925; 1969). The designs of the treatment and the instrument
are difficult because problem solving in terms of restructuring
objects is an abstract theoretical construct, which involves a
complex mental model. In this situation, the think aloud protocol can
be useful to understand the mental construct.
It is important to note that in research the ultimate goal is
concerned with broader concepts rather than a particular software
package or a product created by the software (Yu, 1998). For example,
the evaluator is interested in not only the instructional value of a
Website, but also the concepts of "hypermedia" and "hypertext." Thus,
the refinement of the treatment must align with the intended media
features. More importantly, the media features must be mapped to the
mental constructs to be studied. If the treatment is packed with many
other features that are unrelated to the research objectives, the
subsequent evaluation may be biased.
Conclusion
The differences among treatment effectiveness study, instrument
validation, and performance appraisal may lead to confusion between
the use of test-retest reliability and dependent t-tests for pre-post
difference. Also, these elements may give a false sense of security
in sample size determination when the goals of power and stability
are not differentiated. More importantly, a serious logical fallacy
of circular dependency may result from the failure to rule out rival
explanations. This paper is an attempt to clarify these
misconceptions and to provide practical solutions.
References
- Cronbach, L. J., & Meehl, P. E. (1955). Construct validity
in psychological tests. Psychological Bulletin, 52,
281-302.
- Dickson, C. R. (1975). Role of assessment in behavior therapy.
In P. McReynolds (Ed.), Advances in psychological assessment
(Vol. 3). San Francisco, CA: Jossey-Bass.
- Ericsson, K. A. (1993). Protocol analysis: Verbal reports
as data. Cambridge, MA: MIT Press.
- Ericsson, K. A., & Smith, J. (1991). Toward a theory of
expertise. New York: Cambridge University Press.
- Fan, X. (1998). Item response theory and classical test
theory: An empirical comparison of their item/person statistics.
Educational & Psychological Measurement, 58, 357-384.
- Hurley, P. J. (1988). A concise introduction to logic.
Belmont, CA: Wadsworth Publishing Company.
- Instruction Support Group. (1999) The Conexiones project.
[On-line] Available: http://conexiones.asu.edu.
- Kelley, D. (1998). The art of reasoning (3rd ed.). New
York: W. W. Norton & Company.
- Kohler, W. (1925). The mentality of apes. New York:
Harcourt.
- Kohler, W. (1969). The task of Gestalt psychology.
Princeton, N. J.: Princeton University Press.
- Messick, S. (1980). Test validity and the ethics of
assessment. American Psychologist, 35, 1012-1027.
- Messick, S. (1988). The once and future issues of validity:
Assessing the meaning and consequence of measurement. In H. Wainer
& H. I. Vraun (Eds.) Test validity (pp.33-45).
Hillsdale, NJ: Lawrence Erlbaum Associates, Publishers.
- Potter, C. C. (1999). Measuring children's mental health
functioning: Confirmatory factor analysis of a multidimensional
measure. Social Work Research, 23, 42-55.
- Sechrest, L.; West, S. G.; Philips, M. A.; Redner, R.; &
Yeaton, W. (1979). Some neglected problems in evaluation research:
Strength and integrity of treatments. In L. Sechrest, S. G. West,
M. A. Phillips, R. Redner, & W. Yeaton. (Eds). Evaluation
Studies Review Annual (pp. 15-35). Beverly Hills, CA: Sage
Publications.
- Thompson, B. (1996). AERA editorial policies regarding
statistical significance testing: Three suggested reforms,
Educational Researcher, 25 (2), 26-30.
- Walstad, W. B., & Robson, D. (1997). Differential ite,
functioning and male-female differences on multiple-choice tests
in economics. Journal of Economic Education, 28, 155-171.
- Wolins, L. (1995). A Monte Carlo study of constrained factor
analysis using maximum likelihood and unweighted least squares,
Educational & Psychological Measurement, 55, 545-559.
- Yu, C. H. (1998). Media evaluation. Retrieved from http://www.creative-wisdom.com/teaching/assessment/media.html.
Appendix
Q1: A researcher administers an instrument to a group prior to
the experiment, then he administers the same test again after the
experiment. What kind of statistics should he compute for the test
data?
a. test-retest reliability
b. alternate form
c. dependent t-test for pre-post difference
d. both
Q2: What criteria should a researcher use to determine the
desirable sample size for instrument validation?
a. How likely the null hypothesis can be rejected given that the
null is false.
b. To what degree the response patterns can be stable across
different samples
c. Both
d. None of the above
Q3: Power analysis for determining sample size can be applied
in which of the following situation(s)?
a. Statistical analysis for treatment effectiveness
b. Instrument validation
c. Both
d. None of the above
Q4: Given the statement: "If P then Q," which of the following
is a valid logical deduction?
a. If not Q, not P
b. If Q, then P
c. If not P, then not Q
d. All of the above are valid
Q5: If test scores are high after subjects have been exposed to
a treatment, which is more likely to be true?
a. The treatment enhances learning effectively
b. The test is too easy
c. The subjects are too smart
d. (a) and (b)
e. All of the above
Navigation
Press
this icon to contact Dr. Yu via various channels
|