Contents
IntroductionToday
many instructional programs are delivered through high tech media such
as multimedia, hypertext, World Wide Web, and video conferencing. The
following is a brief outline of procedures for media evaluation.
First of all, you need a very detailed plan. Many people do not plan
ahead, rather they claim that they are doing Exploratory Data Analysis
(EDA). Actually what they do is EDC-exploratory data collection, which
does not exist in any school of research methodology.
This write-up is an attempt to answer some frequently asked questions
and to address several common misconceptions in media evaluation.
Readers are required to have some familiarity with statistical concepts
and procedures. If you do not understand the details, you may try to
underestand the overall framework first, and then fill in the details
later by checking out the books cited in the reference section.
Determine instructional theory
A well-designed instructional package should be theory-laden. It is
important to notice that the mission of media research is not only to
evaluate the product, but also the ideas
behind the product. Five years from now, state of the art technology
that you have presently will be laughable. Technologies just come and
go, but sound instructional theories have a longer life. In a similar
vein, Lockee, Burton, and Cross (1999) made it clear that media
evaluation and media research are fundamentally different:
"Evaluation is practical and concerned with how to
improve a product or whether to buy and use a product. Studies that
compare one program or media against another are primarily evaluation.
Evaluation seeks to find the programs that 'work' more cheaply,
efficiently, quickly, effectively, etc. Research, on the other hand
tends to be more concerned with testing theoretical concepts and
constructs or with attempting to isolate variables to observe their
contributions to a process or outcome." (p.36)
There is nothing new under the sun. The ideas of many high tech
instructional packages could be traced back to traditional pedagogy.
Today some instructional designers conduct research to compare the
effect of static webpages with that of interactive webpages.
Interactivity does not necessarily happen in Web-based instruction
(WBI). In a classroom setting there are one-way lectures and
forum-style discussions. Moreover, today some researchers investigate
the effect of hyperlinking on WBI. Actually, before the age of the
Internet, hyperlinking was implemented and studied on standalone
hypertext systems such as HyperCard and Authorware. Other ideas such as
self-paced learning, collaborative learning, and multimedia learning
could also be found in conventional instructional media.
Therefore, the inferences drawn from media research should go beyond a
particular product or even a particular medium. For instance, the
concern should not be "Can Web-based Instruction enhance learning?"
Rather it should be "Can interactivity, hyperlinking, multimedia,
self-paced learning, and collaborative environment on Web-based
Instruction enhance learning?" Even if later the technology is
outdated, those ideas could be transferred to another medium and the
research findings on those ideas could still carry on as principles and
guidelines for instruction and research
Determine target audience, audience segments, and sample size
- Population and Sample:
Both imagined target audience (population) and actual target audience
(sample) should be defined. The former plays a crucial role in
developing evaluation while the latter is important to the development
of instructional objectives. In order to enhance the generalizability
of the evaluation, it is desirable to obtain two more or samples with
slightly different backgrounds such as engineering majors, business
majors, and humanities majors. Lindsay and Ehrenberg (1993) state that
many researchers focus on how to analyze a single set of data, rather
than how to handle and interpret many data sets. As a result, findings
based upon one sample may not be replicated in another sample and thus
generalization to a broader population is limited. To remediate this
shortcoming, cross-validation within the same sample group or replication of the study with different samples are helpful.
- Audience segments:
Today many high tech instructional media stratifies various audience
segments. For example, a multimedia program comprised predominately of
graphics may be geared toward "visual-oriented learners" while a
text-based program may be tailored for "text-oriented learners." A
linear program may be suitable for "structural learners" whereas a
non-linear hypertext program may be aimed at "exploratory learners."
Some Web-based instruction programs may be targeted at computer novices
while others are designed for computer literates. In most cases, the
same program with different options are delivered to all types of
audiences. In order to evaluate the effect of the program on different
types of learners or the Aptitude Treatment Interaction (ATI),
learners' attributes/aptitudes such as "visual/text-oriented,"
"structural/exploratory-oriented," and "computer literary" should be
clearly defined and discriminant analysis (Eisenbeis , 1972) should be conducted to classify learners.
However, an evaluator may run a risk of ill-defining learners'
attributes and creating an unreliable or invalid instrument resulting
in mis-classification. It is advisable either to use naturally ocurring
(organismic)
types like grade, gender, and race for segmentation, or to adopt
well-defined learner cognitive styles and well-established tests such
as Myers-Briggs Type Indicator (MBTI) to study treatment effect by audience segment (e.g. Jones, 1994).
- Sample size: The sample size should be determined by the power, the effect size, and the alpha level.
- Power is the probability of detecting a true significant difference (Cohen, 1988).
- Effect size is the numerical difference between the
control group and the treatment group in terms of the control group's
standard deviation (Glass, McGraw, & Smith, 1981).
- Alpha level is the cut-off for determining statistical significance.
A larger sample size is not necessarily better than a smaller sample
size if the study is over-powered i.e. When you have a very large
sample, you may prove anything you want but the so-called "significant
difference" is questionable. Nevertheless,
the problem of low power, small effect size, and small effect size is
more common in social sciences than the problem of large sample size.
Proper sample size, power, and effect size should be calculated based
upon the methods introduced by Cohen and Glass.
Define instructional objectives
Objectives drive both instructional design and evaluation. Change in
cognition and change in motivation are two major categories of
instructional objectives:
- Cognition: The cognition aspect is often referred to as the increase of knowledge and skills. Basically, it may involve:
- Rote learning such as memorization of concepts/declarative knowledge (what) and memorization of procedural knowledge (how)
- High level cognitive skills such as logical reasoning (why) and problem solving (why and how)
It is a major pitfall that many evaluators give a one-shot performance
test right after the treatment and draw a conclusion about the
effectiveness of the program from the test. Indeed, a follow-up like
repeated measures is essential due to two reasons:
- Regarding memorization of content material, a good instruction
program should enable learners to retain the information in their long term memory, not just the short term memory.
- Regarding high level cognitive skills, it takes time for
the learners to digest the materials and develop reasoning logics and
problem solving skills. For instance, research competence may be
evaluated a few months after students complete a statistics course.
- Motivation: Many instructional designers expect the effect of ongoing lifelong learning from the students. Essentially this is about motivation. There are two major aspects of motivation, namely,
- Motivation on self: It poses a question like "Could the
instruction enhance the learners' self-image so that they will be
confident enough to take more intellectual challenges?"
- Motivation on subject: It is concerned with "Could
the instruction stimulate their intellectual curiosity on the subject
matter so that they will further their learning through other resources
in the future?"
Depending on the target audience, some instructional programs may
stress the former while others concentrate on the latter. For instance,
one of my colleagues used the World Wide Web as a medium of instruction
for high school drop-outs. For this program ego-building is more
important than acquiring knowledge of the subject matter.
- Mixed Objectives:
The above objectives should be clearly defined so that the researcher
does not measure cognitive skills that are affected by motivation, and
vice versa. For example, it is a common mistake that an instructor
"evangelizes" the merits of a new instructional medium while the
objectives of the instruction are concerned with cognition rather than
motivation.
In this case, the evaluator receives contaminated data and may not know
whether the improvement in performance is caused by the medium, the
instructional design, or by strong motivation i.e. if the students
believe that the treatment can help them, they will try harder to
learn.
Nevertheless, it does not mean that an instructional designer cannot
put both cognition and motivation into the objectives. If both
objectives are included, specific measurement procedures are required
to filter the data.
Define media propertiesThe
instructional designer should ask what specific properties in the
medium can enhance learning. For example, some engineering professors
connect a scanning probe microscope with the Internet to provide an
opportunity of visually exploring and manipulating the subatomic world
in a real time manner. There are at least three features in this
medium:
- Visualization
- Exploration
- Real time manipulation to the real object
Define mental constructs
With reference to cognitive psychology,
the instructional designer should ask what psychological constructs of
the learner will be affected by the above media properties. In this
example, we can map the following changes in mentalities to the
preceding media properties:
- Visual thinking: The learner will develop imagery of the subatomic world and map the images with concepts.
- Exploratory thinking: The learner will adopt exploration as a learning tool and ask "what-if' questions for problem-solving.
- Control: The learner will try to manipulate the subject matter to advance his/her knowledge.
Define physical and behavioral outcomes
The instructional designer should ask how he/she expects the learner to
behave after the above psychological changes occur. These outcomes
should align with the instructional objectives. For instance, those
engineering professors may define the objectives within the cognitive
domain such as "the learner is able to classify various macrostructures
and measure particles by nano-size scale. The tangible outcome would be
"the learner is able to examine the stability and precision of a
microchip in a semiconductor fab."
Develop testable hypotheses
Someone may argue whether it is necessary to develop a hypothesis. A
school of research methodology suggests that no hypothesis should be
pre-determined and the inquiry should be data-driven i.e. let a story
emerges from the data. There is no such thing as "no hypothesis." In
research one can starts with a vague and loose, or clear and form
hypothesis. For example, once a doctoral student insisted that there
wasn't any hypothesis in his study on computer-mediated communication.
Actually, he must at least hypothesized that CMC has certain
instructional values, otherwise, why bother?
Testable hypotheses based upon both mental constructs and physical
outcomes should be formed. A testable hypothesis should be specific
enough and stand a chance to be falisfied. The rationale of hypothesis testing could be explained in the perspective of Principle of Falsification,
introduced by prominent philosopher of science, Karl Popper (1959).
According to Popper, conclusive verification of hypotheses is not
possible, but conclusive falsification is possible. The validity of
knowledge is tied to the probability of falsification. For example, a
very broad and general statement such as "Humans should respect and
love each other" can never be wrong and thus does not bring us any
insightful knowledge. The more specific a statement is, the higher the
possibility that the statement can be negated. If the statement has a
high possibility of falsification and can stand "the trial of fire,"
then we can confirm its validity. Quantification such as the
assertion that "the mean of population A is the same as the population
B" is a high degree of specification. Following the Popperian logic,
the mission of a researcher is to falsify a specific statement rather
than to prove that it is right. Therefore, we test the hypothesis by
attempting to reject it.
The following are some examples of testable/falsifiable hypotheses:
- The learner can understand the subatomic world better if he/she can visualize it.
- The learner can understand the subatomic world better if he/she can explore it.
- The learner can understand the subatomic world better if
he/she manipulate the real object in real time rather than by
simulation.
- The learner can function in a semiconductor fab if he/she understands microstructures through scanning probe microscopy.
The following are examples of untestable/non-falsifiable hypotheses:
- Good instructional design and proper application of media can lead to effective learning.
- Good web design can lead to good use of navigation.
Not only the preceding hypotheses are vague (What is good web design?
What is effective learning?), but also the test results do not carry
any practical value. Welkowitz, Ewen, and Cohen (1982) used a funny
example to illustrate this problem. Suppose a researcher hypothesizes
that college education cultivates students' intelligence. He/she set
the null hypothesis as "The mean IQ of college graduates is 68" and the
alternate hypothesis as "The mean IQ of college graduates is more than
68." No doubt he/she could reject the null hypothesis, but his/her
finding does not contribute anything to educational research. By the
same token, in the first example, the null hypothesis would be "Good
instructional design and proper application of media do not lead to
effective learning." Needless to say, this null hypothesis will be
rejected and the alternate is always right!
The above examples are not falsifiable because they are always right.
But some hypotheses cannot even allow us to find out whether they are
right or wrong. For instance, a Freudian psychologist may use a
smoker's childhood experience to explain his vice: The patient smokes a
lot because he sucked his mother's breasts when he was a baby. But
another patient who was fed by cow milk during his infantry also
consumes a lot of cigarettes now. Then the psychologist said that the
absence of his mother's breasts drives him to seek for compensation
from sucking cigarettes! Instructional psychologists should avoid this
type of untestable theories.
If possible, a researcher should state the alternate hypothesis as a
directional hypothesis for a one-tailed test rather than as a
non-directional hypothesis for a two-tailed test. Compare the following
two hypotheses:
- There is a significant difference between the test scores of the control group and that of the treatment group.
- The test scores of the treatment group is significantly higher than that of the control group.
Needless to say, the first hypothesis is in a "safer" position because
better performance in either the control group or the treatment group
is considered a significant difference. On the other hand, the second
hypothesis is in a riskier position, thereby has a higher probability
of falsification.
Design experimentDesign
of experiments (DOE) is a process to control the environment for
testing variables. To understand how DOE works, one must understand
basic concepts such as factors, levels, within-subjects,
between-subjects, variance control, main effects, and interaction
effects. Although they appear to be simple, I found that they are most
problematic to many people. For instance, when the design should be an
one-way ANOVA with 4 levels, one may misinterpret it as a four-way
ANOVA.
DOE should follow the methodologies recommended by Campbell and Stanley
(1963), Cook and Campbell (1979), and Maxwell and Delaney (1990). To
better conceptualize the design, the evaluator should draw a grid of
the experiment with factors and levels. For example, a basic
experimental design is a pretest-posttest administered to a control
group and a treatment group as the following. This example has two
factors and each factor has two levels.
| Pretest | Posttest
| Control |
|
| Treatment |
|
|
The design can be more complex. For instance, if you want to
discriminate the learner segment, introduce more versions of the
treatment and measure the subjects repeatedly, the grid may look like
the following:
|
| Pretest | Test right after the treatment | Test after a week later
| Control | Visual-oriented learners |
|
|
| Text-oriented learners |
|
|
| Wed-based instruction with dominated text | Visual-oriented learners |
|
|
| Text-oriented learners |
|
|
| Wed-based instruction with dominated graphics | Visual-oriented learners |
|
|
| Text-oriented learners |
|
|
|
In brief, a grid is helpful to visualize the experiment, especially
when you have a complex design with many factors and levels. At the
early development of experimental methodology, quite a few experiments
were applied to argiculture. In those argicultural research, a grid was
used not only in conceptualizing and visualizing the experiments, but
also in the actual implementation. The picture below shows a 5 x 5
Latin square laid out at Bettgelert Forest in 1929. The experiment was
to study the effect of exposure on Sitka spruce, Norway spruce,
Japaneses larch, Pinus contorta and Beech (UCLA Statistics, 1999).
There are software packages for visualizing experimental design. Examples of such software packages are SPSS's Trial Run, SAS's JMP, and SAS's ADX
Besides visualization, these software packages could list all possible
options of experimental design according to your input. The following
figure is an example of a research design made with JMP.
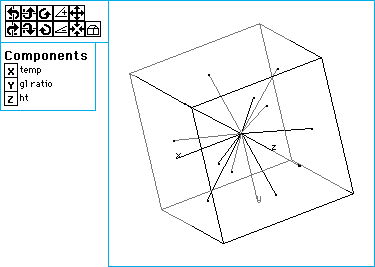
It is very tempting for an evaluator to adopt an extremely complex
design such as a "4 X 4 X 4 X 4 X 4 all wheels drive factorial design"
in attempt to answer all questions. However, the rules of KISS (Keep It
Simple, Stupid) or KISBUTT (Keep It Simple Based Upon T-Tests) should
be applied due to the following reasons:
- In a very complex design the evaluator may eventually find one or
two significant differences out of many variables. If I shoot
continuously with a M16, I can eventually hit someone, of course. In
other words, from a complex model, you may always find some support to
your theory. Thus, it does not have a high degree of falsifiability,
the Popperian principle that was introduced earlier.
- According to the principle of parismony, given
that all other things being equal, a simpler model with fewer variables
is better than a more complex
model. A very complex design such as one involving four-way
interactions may not lead to interpretable results for practical use.
So, keep the design simple with a few variables. However, in the
preceding section it was mentioned that the measurement instrument
should be long enough to achieve high reliability. Are a simple design
with a few variables and a long measurement instrument with many items
contradictory? Not at all. The evaluator could put down 50 items in a
test, but it does not mean he/she will have 50 variables for the final
analysis. For instance, there are hundreds of questions in GRE, but GRE
measures only three mental constructs: Verbal intelligence,
quantitative intelligence, and logical reasoning.
The preceding example is a long instrument with pre-determined mental
constructs. But what if the evaluator is not sure what the underlying
mental constructs are. In this case, he/she could conduct a factor analysis to collapse many items into just a few variables. For more information on factor analysis please consult Comrey and Lee (1992).
Develop measurement instrument
Measurement instruments should be developed for both mental constructs
and physical outcomes. The instrument must comply to the standards of validity and reliability specified by American Psychological Association in Standards for Educational and Psychological Measurement (1985).
The rule of thumb is: Write a long test and run a pilot study
(or several pilot studies). First, the longer the test is, the more
reliable it is. Second, after the pilot study you could throw out
poorly-written items from the long test and retain a shorter version.
Nunnally (1978) suggested that the initial item pool should contain
twice as many items as desired in the final instrument. If you want to
keep the same length, you could replace those bad items with better
items. In brief, if you start with a longer test, you have more options
later. Readers may consult Crocker and Algina (1986) for the details of
test construction. For a summary of the concepts please read my write-up on reliability and validity. For a guideline of the procedure of computing a reliability coefficient, please read my SAS write-up.
Further readings
In 1999, the Board of Scientific Affairs (BSA) of the American
Psychological Association (APA) convened a committee called the Task
Force on Statistical Inference. The mission of this committee is to
develop a guideline of proper practice in psychological research. The
report was published in American Psychologist
and also available online (Wilkinson & Task Force, 1999). Like this
article, the report covers every step of research such as selecting
supporting theories, posting research questions, formulating
hypotheses, designing the experiment, selecting the target population
and sample, measuring the subjects, choosing statistical procedures,
and many others. The suggestions are crystalized from many great
masters in the field. No one will get fired for buying from IBM.
Similarily, no research will go wrong for following APA.
References
American Psychological Association. (1985). Standards for Educational and Psychological Measurement. Washington D.C.: Author.
Campbell, D. & Stanley, J. (1963). Experimental and quasi-experimental designs for research. Chicago, IL: Rand-McNally.
Cohen, J. (1988). Statistical power analysis for the behavioral sciences. Hillsdale, N.J. : L. Erlbaum Associates.
Comrey, A. L., & Lee, H. B. (1992). A first course in factor analysis. Hillsdale, N.J. : L. Erlbaum Associates.
Cook, T. D., & Campbell, D. T. (1979). Quasi-experimentation: Design and analysis issues for field settings. Boston, MA: Houghton Mifflin Company.
Crocker, L. M., & Algina, J. (1986). Introduction to classical and modern test theory. New York : Holt, Rinehart, and Winston.
Eisenbeis, R. A. (1972). Discriminant analysis and classification procedures: theory and applications. Lexington, Mass., Heath.
Glass, G. V., McGraw, B., & Smith, M. L. (1981). Meta-analysis in social research. Beverly Hills, Calif. : Sage Publications.
Jones, P. (1994). Computer use and cognitive style. Journal of Research on Computing in Education, 26, 514-522.
Lindsay, R. M., and Ehrenberg, A. S. C. (1993). The Design of Replicated Studies, The American Statistician, 47, 217-228. Lockee,
B. B.; Burton, J. K., Cross, L. H. (1999). No comparison: Distance
education finds a new use for 'no significance difference.' Educational Technology Research and Development, 3, 32-42.
Maxwell, S. E., & Delaney, H. D. (1990). Design experiments and analyzing data: A model comparison perspective. Belmont, CA: Wadsworth Publishing company.
Nunnally, J. C. (1978). Psychometric theory (2 nd ed.). New York: McGraw-Hill.
Popper, K. R. (1959). Logic of scientific discovery. London : Hutchinson.
UCLA Statistics. (1999). History of statistics. [On-line] Available URL: http://www.stat.ucla.edu/history.
Welkowitz, J., Ewen, R. B., & Cohen, J. (1982). Introductory statistics for the behavioral sciences. San Diego, CA: Harcourt Brace Javanovich, Publishers.
Wilkinson, L, & Task Force on Statistical Inference. (1999).
Statistical methods in psychology journals: Guidelines and
explanations. American Psychologist, 54, 594?04. [On-line] Available URL: http://www.apa.org/journals/amp/amp548594.html
Navigation
Index
Simplified Navigation
Table of Contents
Search Engine
Contact
|